Massive Geospatial Data Storage and Processing for Intelligent and Sustainable Urban Transportation (MaGIST)
Urban mobility and its environmental impact is a problem of great concern for citizens. In recent years, great efforts have been made to provide solutions, especially within the scope of Intelligent Transport Systems. In addition, the generation of data in this area has grown, largely thanks to the proliferation of sensing systems and the involvement of citizens through mobile technologies. Among the data collected we can highlight data on urban infrastructure (road network, public transport networks, etc.), data from fixed sensors (capacity stations, air particle sensors, etc.), from mobile or on-board sensors (such as location of the urban bus or bicycle fleet), of mobile mapping systems (scanners based on LiDAR technology, cameras, and navigation systems, etc.), or citizen activity data (information provided voluntarily in applications of mobility, comments on social networks, etc.).
Part of the efforts has been directed to the collection of a large volume of data, of a great variety of types, that reflect both the structural and the present state of the city with veracity, and that change with great velocity. New technologies in the field of Big Data take on a fundamental role in this new type of solutions, and important challenges arise in the collection, storage, analysis and publication of these large amounts of data.
On the other hand, efforts have been directed to define models, algorithms and visualization techniques to obtain relevant information for stakeholders such as city service managers, citizens, the productive sector or higher levels of the public administration. These solutions include traffic flow analysis techniques, observation and prediction of air quality, multimodal route calculation applications that combine walking routes with different types of transport, and support systems for maintaining infrastructures. For the implementation of these solutions, machine learning, stream processing, approximate querying, visual analytics, exploratory analytics and other techniques are used.
The variety and volume of the data, the veracity necessary for them to be useful, the velocity at which they are generated, as well as the variety of data modeling, processing and visualization techniques make information management in the urban environment a paradigmatic problem of Big Data. However, the most common situation in today's cities is usually a heterogeneous and little cohesive set of data and tools.
The main objective of the MaGIST project is to define the architecture of a system for intelligent and sustainable urban transport with contributions in the common layers of any information system: data collection, storage, processing, analysis and visualization of information. The achievement of this objective is aligned with the challenge of intelligent, sustainable and integrated transport, addressing the development of a system that allows planning an efficient use of resources, strengthening accessibility and functional integration, preserving the environment and improving the quality of citizens' life.

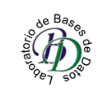
MaGIST-SAVES: Streaming and analytic visualization and exploration of spatial big data
PID2019-105221RB-C41
+ info
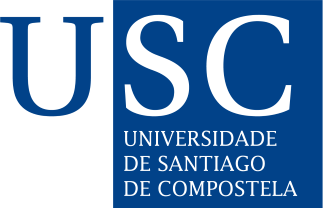
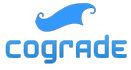
MaGIST-ELA: Large Scale Geo-processing for Exploratory and Learning Analytics
PID2019-105221RB-C42
+ info
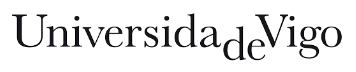
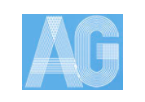
MaGIST-ERIUM: Geospatial Intelligence for improving decision-making in Urban Mobility
PID2019-105221RB-C43
+info

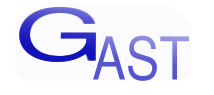
MaGIST-RALES: Real Time Social Sensor Analysis and Deep Learning based Resource Estimations for Multimodal Transport
PID2019-105221RB-C44
+info